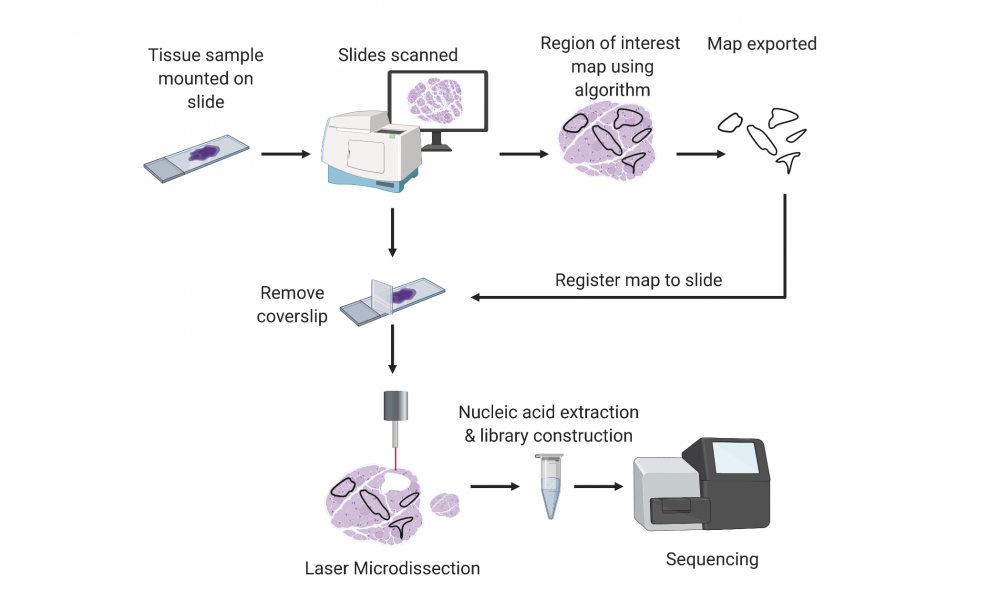
As researchers work towards establishing whole genome and transcriptome analysis into clinical practice for cancer care, scientists at the GSC continue developing innovative laboratory methods to improve efficiency and accuracy.
In a new study, published in the Journal of Pathology, led by GSC Director and Distinguished Scientist Dr. Marco Marra and the GSC’s Instrumentation Group Leader Dr. Robin Coope, researchers used machine learning algorithms to develop an automated method for tumour sample processing, circumventing the need for expert pathologist review and reducing processing time.
The procedure was developed as part of the Personalized OncoGenomics (POG) program at BC Cancer, a precision oncology initiative that performs whole genome and transcriptome sequencing to identify targetable genetic alterations in patients with metastatic disease.
Tumour sequencing usually relies on biopsies—small tissue samples taken from a patient’s tumour. During the procedure, it is impossible for the surgeon to ensure that the biopsy contains only cancerous tissue, and as a result, tumour biopsies consist of a mixed population of cancerous and healthy cells. To improve the quality of tumour sequencing, biopsies can be dissected to enrich the sample for tumour cells, reducing the amount of contaminating healthy tissue.
Sample enrichment is often performed using a technique called laser capture microdissection (LCM), a widely used method that employs a microscope with a laser to dissect thin slices of tissue adhered to a glass slide. LCM instruments are designed to dissect very small patches of tissue and in some cases, even single cells. To ensure sufficient tumour material is dissected for genomic analysis, the tumour sections must be manually identified to produce a map, which is then used by the LCM instrument for microdissection of the entire slide.
To automate slide annotation, the team used tissue slides that were first annotated by expert pathologists to train a machine learning algorithm to distinguish cancer cells from healthy cells and to produce a dissection map that could be used for LCM. They put their method to the test and found that, following dissection, high quality nucleic acids could be extracted in quantities sufficient for whole genome and transcriptome sequencing, and that the dissection successfully increased the tumour cell content in the sample.
“LCM has been employed in research for many years to extract small groups of cells or even single cells to study their genomic characteristics,” says Dr. Coope. “Our approach automates the identification and boundary mapping of tumour cells across a whole slide, so thousands or tens of thousands of cells can be dissected in one step.”
This proof-of-concept study provides the foundation for further innovation and method development. The team is currently running tests to ensure the method is applicable to a wide range of tumour types and slides prepared by different laboratories. Recently, they have employed a different laser and specially coated slides to reduce the dissection time from approximately 40 minutes to just one minute per slide.
“The POG program aims to pave the way for genomic analysis to become part of routine cancer care,” says Dr. Coope. “Automated LCM has the potential to improve the efficiency and accuracy of tumour sequencing and do so in a way that can be scaled and expanded to meet the needs of a broader patient population.”This work was supported Genome BC, Genome Canada and the Canada Foundation for Innovation.
Robin JN Coope, Colin Schlosser, Richard D. Corbett, Stephen Pleasance, Basile Tessier-Cloutier, Pawan Pandoh, Heather Kirk, Simon Haile, Yongjun Zhao, Andrew J Mungall, Marco A. Marra. 2020. Whole-Slide Laser Microdissection for Tumour Enrichment. Journal of Pathology. Online ahead of print.
Learn more about the Digital Pathology Project
Learn more about the Personalized OncoGenomics program
Learn more about research at the GSC